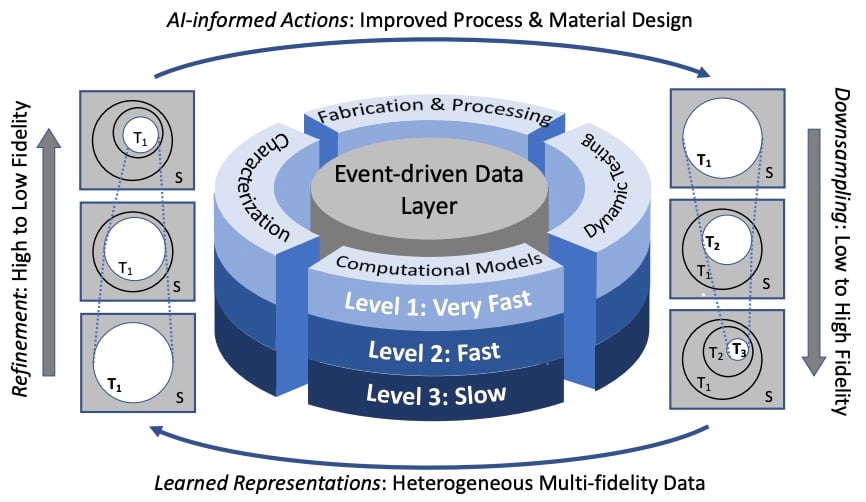
Leveraging the capabilities established by the Center for Materials in Extreme Dynamic Environments (CMEDE) and the AI for Materials Design (AIMD), AI-Driven Integrated and Automated Materials Design for Extreme Environments (AMDEE) is a three-year ARL-funded cooperative agreement that focuses on AI- and data-driven materials design. AMDEE is expanding the current hardware capabilities in AIMD to generate high quantities of valuable application-relevant data (e.g., by incorporating integrated thermal/mechanical laser shock, novel ultraviolet microscopy, and a broader range of materials – RMPEA’s and light alloys). The basic robotic automation developed in AIMD will be extended to reach more stations and demonstrate a new level of sophistication in applying materials and processing data. Most importantly, this project focuses on developing and implementing machine learning and AI tools, not only to evaluate materials, but to make actionable design decisions in the context of an automated system, as a large step towards an autonomous materials design capability.
AMDEE activities will tackle the challenges associated with data- and AI-driven design of materials in extreme conditions and is organized around five key AI needs:
- Significant quantity of coordinated materials data relevant to extreme environments;
- Efficient prediction of the future ramifications of various decisions;
- Reliable approaches to identify optimal decision(s);
- Actionable response to the decision(s);
- Event-driven integration of data to connect the design, decision, and control loops.
This research project will answer the critical question of which alloys exhibit optimal high temperature and high-rate properties, leveraging high-throughput fabrication, characterization, and testing of materials in extreme environments in an AI-driven decision framework. As a result, this research will have significant impact on the materials research community, as well as in applications of particular importance to the U.S. Army, including soldier protection, ballistics, and hypersonics.
Principal and Co-Investigators
Acknowledgement Statement
Research was sponsored by the Army Research Laboratory and was accomplished under Cooperative Agreement Number W911NF-23-2-0062. The views and conclusions contained in this document are those of the authors and should not be interpreted as representing the official policies, either expressed or implied, of the Army Research Laboratory or the U.S. Government. The U.S. Government is authorized to reproduce and distribute reprints for Government purposes notwithstanding any copyright notation herein.