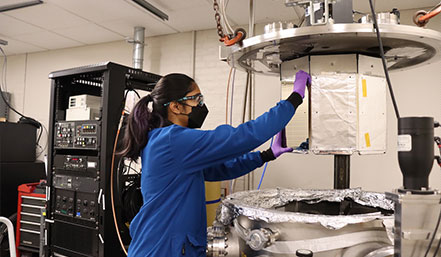
A simulation of the robot operating system picking up a sample andplacing it into an AIMD component. This simulation assists in designing the trajectory for each UR10e robot arm within the system. Gazebo software and open dynamics engine library data was used to create this simulation.
A simulation of multiple UR10e robot arms within the overall robot operating system. This simulation assists in synchronizing the movement of the robot arms and demonstrates their reach. Gazebo software and open dynamics engine library data was used to create this simulation.
Artificial Intelligence for Materials Design (AIMD) is a major research project laboratory that integrates multiple tasks focused on high-throughput and machine learning (ML)-driven materials development. A centrally controlled robotic automation system links high-throughput characterization and dynamic testing, all driven by ML and computational modeling, and AI-guided decision-making. This laboratory provides a proof of concept for high-throughput fabrication, characterization, and testing of materials in extreme environments. While the framework of this capability can be applied to a variety of materials designs questions, the current laboratory addresses the critical research question of which alloys exhibit optimal dynamic properties. As a result, AIMD will have a significant impact on U.S. Army material applications and on the broader materials research community.
Specific research tasks in AIMD include:
- Integrative AI-driven alloy design and coordination
- Machine learning and computational modeling
- Alloy fabrication and processing via sputter deposition
- Sample preparation and high-throughput characterization via XRD/XRF and profilometry
- High throughput laser shock to determine spall strength, HEL, equation of state
- Robotic automation for integrated high-throughput experimentation
Principal and Co-Investigators
Acknowledgement Statement
Research was sponsored by the Army Research Laboratory and was accomplished under Cooperative Agreement Number W911NF-22-2-0014. The views and conclusions contained in this document are those of the authors and should not be interpreted as representing the official policies, either expressed or implied, of the Army Research Office or the U.S. Government. The U.S. Government is authorized to reproduce and distribute reprints for Government purposes notwithstanding any copyright notation herein.
Questions about the data management system on AIMD should be directed to [email protected].