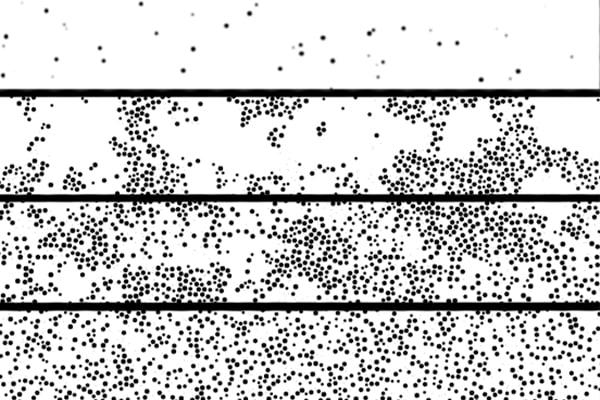
MLM – ML-augmented Physics-based Models
HT-MAX hypothesis: ML tools will enable predictive physics-based models of materials in extreme environments by providing efficient linkages between relevant scales and multiple physics.
MLM Objectives
- Provide mechanistic insights into experimental observations of the dynamic material performance at a range of temperatures;
- Develop novel predictive models for the response of materials under dynamic thermo-mechanical loading, for compositions and microstructures of interest; and
- Augment the HT-MAX dataset with models built on statistically significant numbers of microstructure instantiations.
Molecular scale modeling
- MD models for characterization:
- Fundamental properties
- Nanoindentation
- MD models related to shock and spall:
- Hugoniot curves
- Spall failure
- MD/continuum bridging
- Dim reduction, field-field mappings
Linking to continuum and continuum-scale modeling
- Scale-bridging MD to continuum
- High-throughput simulation of laser shock
- Reduced-order models of spall
- Lower-scale representations
- U-net mappings
- Uncertainty quantification
- Micro- to macro-scale models
- Links to application