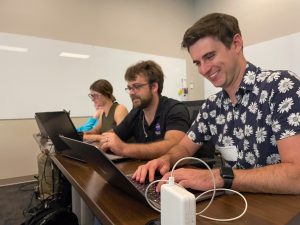
Workforce development is a cornerstone of the MSEE URA.
The Materials Science in Extreme Environments University Research Alliance (MSEE URA) features collaborators from all kinds of backgrounds. As a basic research consortium, workforce development is a key driver of the MSEE URA. Many MSEE participants are students or postdoctoral researchers.
In the first week of September, MSEE held two events for their graduate students and postdocs in support of the alliance’s workforce development goals. Both events took place at Eglin Air Force Base (AFB) in Florida, where attendees were able to network and explore the facilities and career opportunities available at an Air Force Research Laboratory (AFRL).
The first of these events was the second annual Student Symposium. On September 5 and 6, attendees of the student-organized symposium participated in a full slate of networking, mentorship, and educational opportunities.
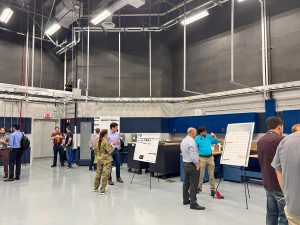
18 of MSEE’s graduate students and postdocs traveled to Eglin Air Force Base in Florida for the second annual Student Symposium. The event included research presentations, networking opportunities, and tours.
The symposium’s first day featured a discussion with Kyle Overdeep, an AFRL technical program manager. Overdeep, a former DTRA-funded graduate student, discussed his journey from PhD student to technical program manager. After hearing from Overdeep, students presented their MSEE research through oral talks and a poster session, followed by tours of some of the facilities within the Advanced Munitions Technology Complex at Eglin AFB.
Day two of the symposium continued with a networking session and tours of the Terminal Engagement Research Facility and the McKinley Climatic Laboratory. Throughout the symposium, MSEE students and postdocs were able to engage with AFRL researchers and scientists, including the Branch Chief of the Energetics Materials Branch, Jacob Morris.
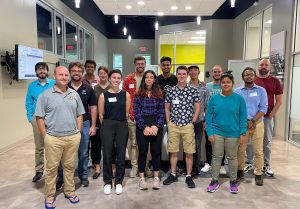
The Uncertainty Quantification Short Course, led by instructors Dimitris Giovanis and Dimitrios Tsapetis, took place after the Student Symposium.
Following the student symposium, AFRL researchers and MSEE students and postdocs participated in a two-day short course on uncertainty quantification (UQ) in physics-based modeling using Python. The UQ Short Course, led by Dimitris Giovanis and Dimitrios Tsapetis of Johns Hopkins University, was held at AFRL’s off-site facility, the Doolittle Institute.
Participants in the short course were introduced to uncertainty quantification (UQ) in computational modeling using the Python programming language and a variety of UQ methods, including forward propagation of uncertainty, surrogate modelling, and Bayesian inference using the UQpy toolbox.